Consumer Tipping Point: Initial Building Blocks
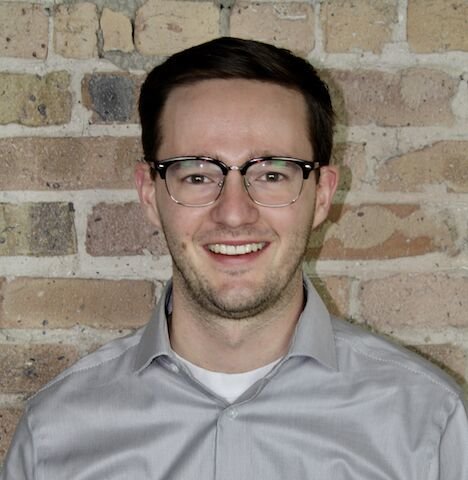
Business Intelligence Analyst, Fooda
This post comes from Eric Ward, a Business Intelligence Analyst for Fooda’s Data Science and Analytics team. He came into the newly-created role to kick off the effort of building out the enterprise reporting environment for Fooda. He hates stacked bar charts, but loves calling everyone super bud.
One challenge the Fooda Data Science and Analytics team is working towards is to able to pinpoint the exact moment when a consumer internalizes the value proposition of the product we provide. Fooda labels this as the “Tipping Point”. This is no easy feat, and depends on hours of data consolidation, investigation and banging your head against the wall while encountering dead ends.
While there are numerous topics to cover with regards to identifying the consumer Tipping Point, below covers one of the essential building blocks to begin to understand how and why consumers behave the way they do.
Consumer Lifetime Value (CLV) can be defined as the entire future purchasing value of an individual within your consumer population. We view CLV as an essential building block of Tipping Point because it helps define the health of our consumer population. Regularly measuring CLV can ensure that we are meeting the needs of our consumer base and look for ways to increase consumer retention.
You’ll find dozens of methodologies online on the best ways to calculate CLV. Many companies fall into a trap by taking a more simplistic approach hoping that it gets “close enough” to make decisions around consumer acquisition costs and expected retention.
Many of these models follow a broad approach of calculating the average net revenue for the population (how much we make vs. how many people buy from us) and multiplying it by some expected lifetime. These models look at the population as a whole, therefore give a generic number of what to expect out of an average consumer, but it won’t let you segment your populations based on behaviors. Not all consumers follow the same pattern of behavior, and therefore should not have the same lifetime value.
To explain this point, please review the diagram below. Which of these consumers would you believe have a high probability of purchasing from you again?
My guess is that you would at least say Teddy and Kim, but hopefully you also said Jerry. We intuitively say these three people based on the fact that Teddy purchased recently, Kim is a new customer with three quick purchases, and Jerry has demonstrated a consistent buying pattern.
While all four of the consumers have three purchases at this point, they most likely will not continue to purchase at the same pace. The question then becomes, how many purchases can I expect from a given consumer over the next 6 months, 12 months, 18 months? To address these questions, Fooda leverages a methodology from a white paper titled “‘Counting Your Customers’ the Easy Way: An Alternative to the Pareto/NBD Model” as well as an open source Python package, Lifetimes, which is supported by the Shopify data team.
This methodology takes a mathematical and probabilistic approach to analyze the past behaviors of a consumer along with their population pool in order to predict the likelihood an individual will purchase again and subsequently calculate CLV for that individual. The methodology focuses on the following three crucial points to build out a model to understand an individual and predict future behavior:
Being able to back into these three metrics for each consumer is feasible as long as the individual transactional level data is available and can tie closely to a consumer within the population.
With these three points, the transactional history of an individual and the population can be analyzed to model a consumer’s journey through their lifetime. This model can be used to forecast future purchases, hypothesize the likelihood of a consumer still being “alive” (continuing to purchase, not actually dead), and segment the population into individuals with similar buying behavior.
Fooda’s CLV model is automated in an Airflow pipeline using a series of Python and SQL scripts to serve a centralized data warehouse built out in Redshift. The automated model pushes forecasted purchases and likelihood of future purchases back into Fooda’s Data Warehouse to be tied to additional attribution. The additional attribution gives us the ability to dive into deeper Tipping Point analysis, reporting, and segmentation of the consumer base.
While Consumer Lifetime Value is only one aspect of understanding the consumer population, it is an essential piece of the puzzle to better understand and serve our consumers to optimize the consumer experience with our products.